صفحه اعضا هیئت علمی - دانشکده علوم ریاضی و کامپیوتر
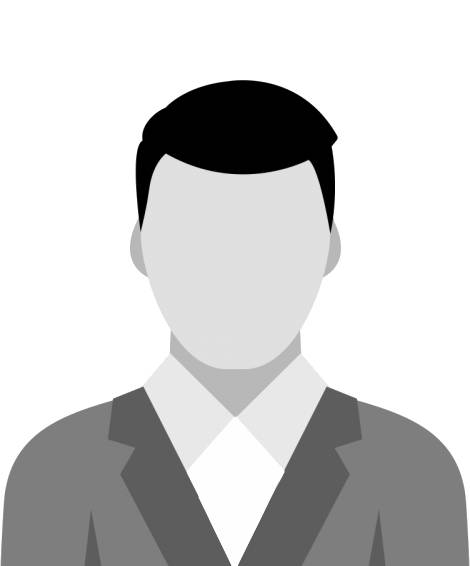
Associate Professor
Update: 2025-03-03
BEHZAD MANSOURI
دانشکده علوم ریاضی و کامپیوتر / گروه آمار
Master Theses
-
مطالعه روش¬ STR برای تجزیه سری های زمانی
علاء فائق احمد 1403 -
برآورد ناپارامتری تابع توزیع تجمعی شرطی و توابع چندكی با آمیخته ای از متغیرهای رسته ای و پیوسته
سنا عبیاوی 1402 -
روش های هموارسازی نمایی خودگردان سازی انباشته با استفاده از تجزیه STL و تبدیل باكس- كاكس
علی عیدان مهدی 1402 -
برآورد تابع توزیع تجمعی چند متغیره با استفاده از روش کرنل
شیوا ثابت 1401 -
پیش بینی سری های زمانی با الگوهای فصلی پیچیده با استفاده از هموارسازی نمایی
رقیه شجیراتی 1400 -
برآورد چگالی های مفصل با استفاده از تعمیم روش کرنل- تبدیل
سارا حاجی شرفی 1399 -
تصحیح اریبی مرزی برآورد ناپارامتری تابع مفصل و کاربرد آن در تحلیل وابستگی بین قیمت نفت و شاخص سهام در ایران فاصله سال های 1397-1390
مصطفی جماشیان 1398 -
مطالعه نمونه هایی از چهار رویکرد رها از مدل، مدل محور، مبتنی بر پیچیدگی و پیش بینی محور در خوشه بندی سری های زمانی
سحر مرادی 1397 -
برآورد تلاطم در داده های قیمت طلا در فضای حالت با استفاده از الگوریتم متروپلیس هستینگ ذرات
اعظم عادلی پور 1397 -
روش تصحیح اریبی ضربی با استفاده از کرنلهای نامتقارن برای برآورد چگالی ناپارامتری دادههای نامنفی
لیلا رجبی 1396The probability density function is a fundamental concept in statistics which explain the random behavior of a physical phenomenos. In statistics, without knowing the probability density function, itʼs not possible to deduce the population charactirstic from a given random sample. Determining the distribution of estimators is not simply feasible. In order to achieve the density function, parametric and nonparametric approach can be used. In nonparametric estimation, the density function f is unknown, and in this case, the data must determine the s estimate. The advantage of nonparametric methods is that the data is free from the constraints created by parametric methods and can express all its features. There are various methods for nonparametric density estimation. The kernel estimator with symmetric kernel functions is a common and efficient method for estimating density in a nonparametric method. However, when dealing with strongly positive data, this estimator has a boundary effect, which means that the estimated bias in points near the boundary is far from the points far apart. One of the new methods for bias correction when dealing with the boundary effect is asymmetric kernels, which are consistent with the density under estimate. In this thesis, various approaches to dealing with the boundary effect when proposed by various researchers are reviewed. In addition, two classes of multiplicative bias correction (MBC) methods are analyzed in use of asymmetric kernels as a boundary effect correction method and the performance of these two classes of estimators are compared with the other bias correction estimators.
-
آستانه سازی دوتایی در برآورد تابع با استفاده از موجک
زینب محمدعلی پاره چی 1395In nonparametric regression, smoothing techniques could be used in order to remove noise from a signal and signal estimation. There are various methods, such as kernel, spline, wavelet …, to smooth a collection of data. However, in terms of risk optimization, wavelet method is the best among other existing methods. Wavelet smoothing technique known as wavelet shrinkage methods. Nonlinear wavelet estimators through thresholding was firstly introduced by Donoho and Johnstone (1994) in nonparametric regression. In order to choose appropriate threshold value, several estimators have been suggested by researchers. The estimates belong to a wide range of Classical and Bayesian methods. In this thesis, Classical methods include FDR, CV, Universal and CMWS; Bayesian methods includes CEB-Mean Kill and E-Bayes thresh; Block methods includes Visu Shrink, Sure Shrink and Sure Block; and two algorithms BABTE and BITUP for Bivariate thresholding, have been investigated. In simulation study, the BABTE algorithm of bivariate thresholding, performs better than other methods. At the final chapter of thesis, Iranian light oil data on a monthly basis from November 1991 to February 2013 were analyzed. The smoothing effect on modeling and forecasting of two models in Box-Jenkins class and Exponential smoothing class, were investigated.
-
هموار سازی برآورد طیف موجک تکاملی با استفاده از انقباض موجکی بیزی
نرگس حسن زاده 1394A time series can be modelled by a locally stationary wavelet (LSW) process using the estimation of the associated evolutionary wavelet spectrum (EWS). We can estimate the evolutionary wavelet spectrum by wavelet periodogram. The raw wavelet periodogram is a biased and nonconsistent estimator of the EWS. The wavelet periodogram needs to be smoothed to obtain consistency. For smoothing wavelet periodogram, we can use wavelet shrinkage methods. In this methods assumes that the noises have normal distribution. In this thesis, we use the Bayesian Wavelet Shrinkage and translation-invariant methods for smoothing wavelet periodogram.The results show that the Bayesian Wavelet Shrinkage have good performance.
-
آزمون مانایی بر اساس موجک و فواصل اطمینان تقریبی برای اتوکواریانس ها در سریهای موضعی مانا
عیدی شهنی - بهاره 1393Checking time series stationarity is among the first and most basic steps in the analysis of time series. Testing second-order stationarity assumptions is therefore of great importance. Since there are many different types of nonstationarity in time series, so we need variety of test in order to check stationary assumptions. So far, various methods for testing time series second-order stationarity, have been studied by researchers, that some of them consider mean function and some others consider autocovariance function. Some of these tests are designed in the time domain and some others are based on spectral properties in frequency domain. Wavelets are appropriate tools for nalysis nonstationary time series due to their time-scale local properties
In this thesis, we review various tests for second-order stationarity of time series. Also, we develop approximate confidence intervals for time-varying autocovariance of locally stationary time series based on wavelets. Then we illustrated the performance of various methods with simulated data. We also analysis real data sets including seismic data and Iran Khodro’s shares returns and compute the confidence interval for its local autocovariance.
-
ممیزی سری های زمانی با استفاده از تقریب معیارهای کولبک – لیبلر و چرنوف در حوزه موجک ها
عباس نژاد-میترا 1393Kullback - Leibler and Chernoff information measure have been well developed for stationary time series. In recent decades, wavelet theory extended in many scientific ground. The main objective of this thesis is extending Kullback-Leibler and Chernoff information measures to provide appropriate criteria for discrimination between both stationary and non stationary time series. Kullback - Leibler and Chernoff criteria have been approximated using discrete wavelets transform for discrimination stationary time series. However, in many cases, we faced with discrimination of procedures that are not necessarily stationary. Long memory procedures are important class of non stationary time series. we also investigate the problem of discrimination in this class of time series.Then we show the performance of our approximations using both simulated and real data set. The results indicate low misclassification rate related to our proposed criteria.
-
بررسی همبستگی متقابل دو سری زمانی نا ایستا با استفاده از فرآیندهای موجکی ایستای موضعی
اعظم بهبهانیان 1392